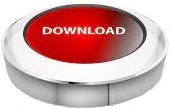
However, storing sufficient examples from all previous tasks may be unreasonable in systems with limited resources or long lifetimes. Mnih et al., 2013) and has been studied in the lifelong learning literature (Isele and Cosgun, 2018 Rolnick et al., 2019 Hayes et al., 2021). The technique of experience replay of past data is common in deep RL (e.g. One strategy for managing experiences is to recall data from previous tasks and mix it with data from the current task when training, essentially transforming sequential LRL into batch multi-task reinforcement learning (RL) (Brunskill and Li, 2013). One approach to meet the challenges of deep LRL is by careful managing the agent’s learning experiences, in order to learn (without forgetting) and build internal meta-models (of the tasks, environments, agents, and world). Deep neural networks are especially prone to instability, often exhibiting catastrophic forgetting of previous tasks when trained on multiple tasks that are presented sequentially (Kirkpatrick et al., 2016). : learning the current task while maintaining performance on previous tasks. Lifelong Reinforcement Learning (LRL) involves training an agent to maximize its cumulative performance on a stream of changing tasks over a long lifetime. Performance than the types of replay used. That the architecture of the sleep model might be more important for improving Promising approach that pushes the state-of-the-art in GR for LRL and observe Well-known architecture for class-incremental classification) is the most
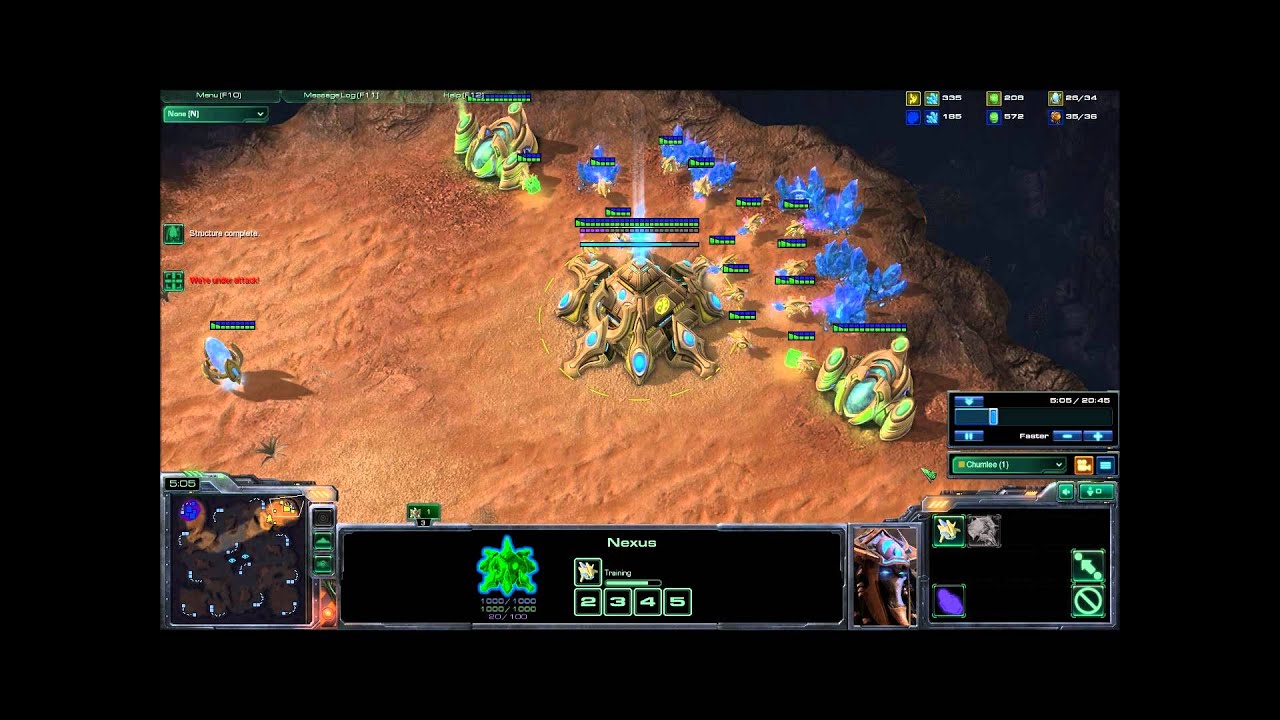
We find that a small random replay buffer significantly We also show improvements in established lifelong GR prevents drift in the features-to-action mapping from the latent vector Performance wrt task expert, and catastrophic forgetting. Learning, generalization to unseen tasks, fast adaptation after task change, Impact of the design choices on quantitative metrics that include transfer We report several key findings showing the Proposed algorithms on three different scenarios comprising tasks from the Naïve GR and adding ingredients to achieve (a) and (b). We study three deep learning architectures for model-free GR, starting from a
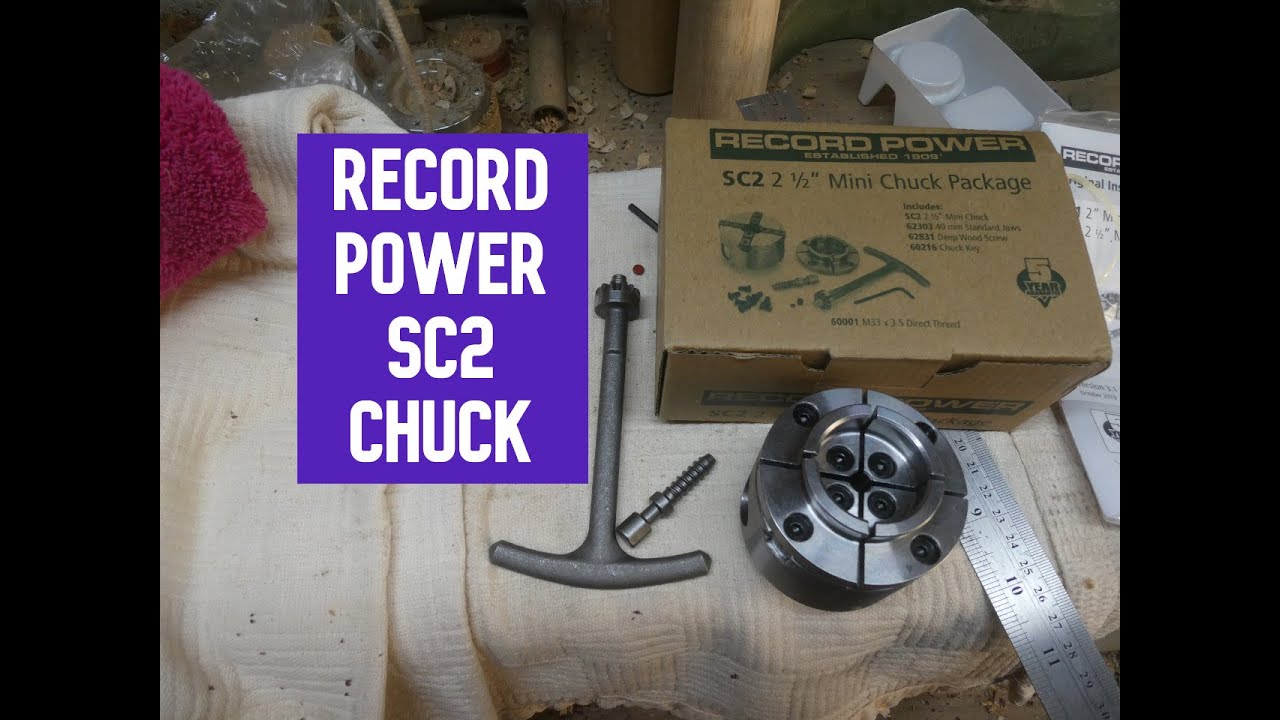
Learned using deep RL, and (b) Model-free end-to-end learning. Introspective density modelling of the latent representations of policies Present a version of GR for LRL that satisfies two desiderata: (a) Inspired replay mechanism that augments learning experiences with self-labelledĮxamples drawn from an internal generative model that is updated over time. (without forgetting) and build internal meta-models (of the tasks,Įnvironments, agents, and world). (LRL) is careful management of the agent's learning experiences, to learn One approach to meet the challenges of deep lifelong reinforcement learning
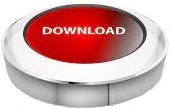